ClipRover: Zero-shot Vision-Language Exploration and Target Discovery by Mobile Robots
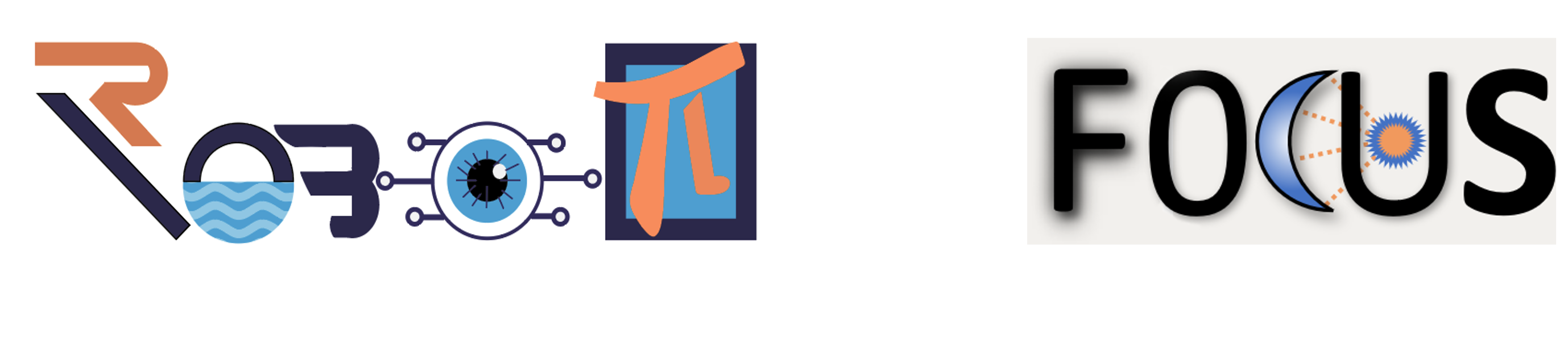
Overview

Vision-language navigation (VLN) has emerged as a promising paradigm, enabling mobile robots to perform zero-shot inference and execute tasks without specific pre-programming. However, current systems often separate map exploration and path planning, with exploration relying on inefficient algorithms due to limited (partially observed) environmental information. In this project, we present a novel navigation pipeline named ClipRover for simultaneous exploration and target discovery in unknown environments, leveraging the capabilities of a vision-language model (VLM) named CLIP. Our approach requires only monocular vision and operates without any prior map or knowledge about the target. The VLM framework adopts a modular architecture organized into three key stages: perception, correlation, and decision. This modular design enables the decomposition of complex system-level challenges into smaller, manageable sub-problems, allowing for iterative improvements to each stage. For comprehensive evaluations, we design the functional prototype of a UGV (unmanned ground vehicle) system named Rover Master, a customized platform for general-purpose VLN tasks. We integrate and deploy the ClipRover pipeline on Rover Master to evaluate its throughput, obstacle avoidance capability, and trajectory performance across various real-world scenarios.