Light attenuates exponentially underwater with propagation distance due to two physical characteristics of light: scattering and absorption. Forward scattering is responsible for blur whereas backscatter causes contrast reduction. Besides, light absorption by water has a prominent spectral dependency, which depends on the specific optical properties of a waterbody, distance of light sources, salinity, and many other factors. These physical characteristics are represented by the underwater image formation model, which, in recent years, have been successfully applied for image restoration and scene rendering tasks.
Our research focuses on the problems of image restoration or enhancement - with the objective of ensuring robust perception by underwater robots. Recently, we have been exploring style transfer, detailed scene understanding, and 3D reconstructions as well. To this end, we investigate both physics-based models and learning-based solutions to advance the state-of-the-art.
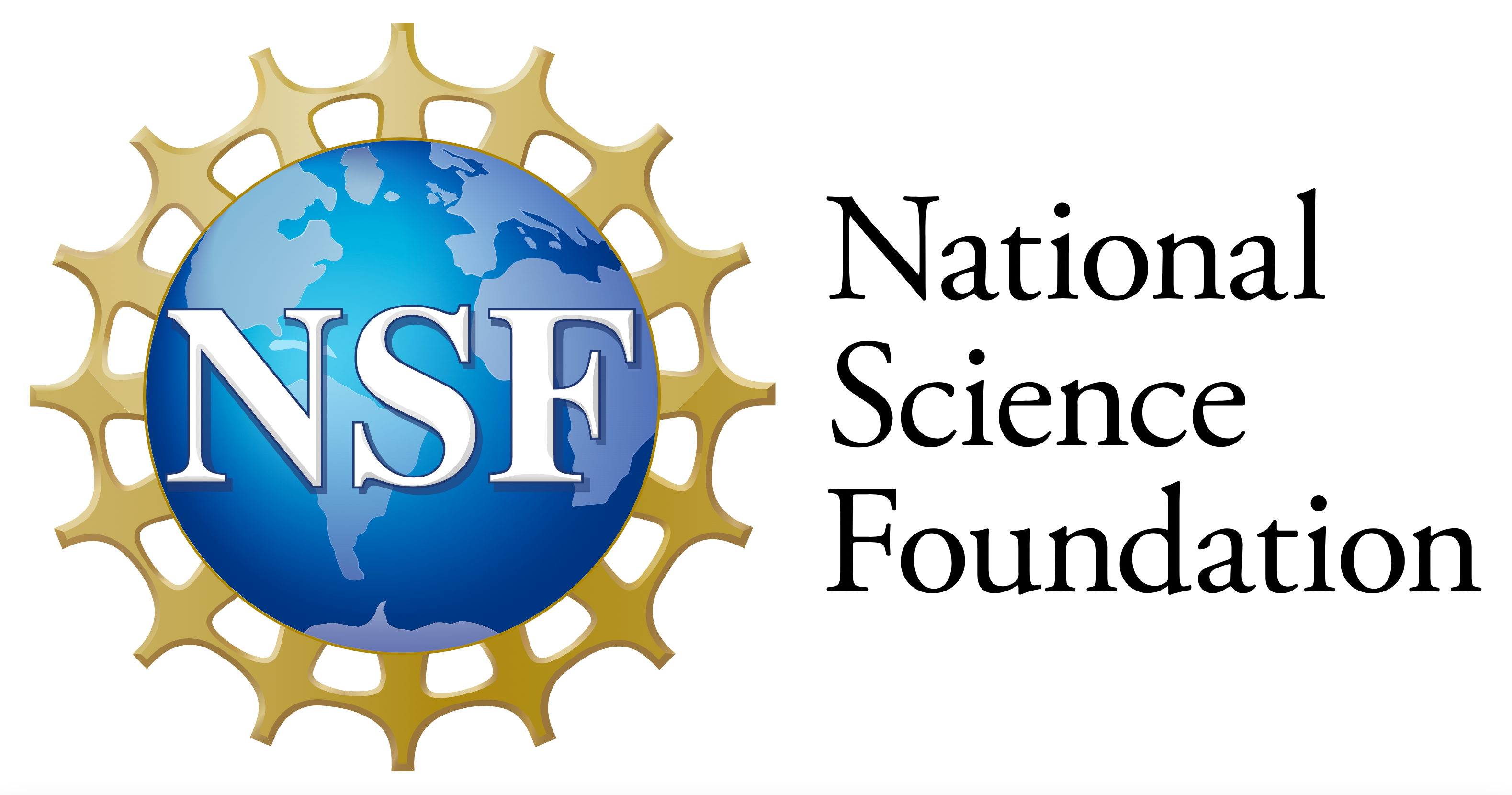
AquaFuse Project
We introduce the idea of AquaFuse, a physically accurate method for synthesizing and transferring waterbody style in underwater imagery. AquaFuse leverages the physical characteristics of light propagation underwater for synthesizing the scene into object content and waterbody. Subsequently, the decoupled object content is rendered into other waterbody types for generating different scenes with the same object geometry. It is a new technique for domain-aware data augmentation by fusing waterbody across different scenes. The traditional approaches rely on perspective or isometric constraints (rotations, translations, scaling) or altering basic photometric properties (brightness, color, contrast). We hypothesize that physically accurate waterbody fusion can be an effective way to augment data for underwater image recognition and scene rendering research. Coming Soon
Domain Projection from RMI Space
In our UDepth Paper we introduced RMI Input Space by exploiting the fact that red wavelength suffers more aggressive attenuation underwater,
so the relative differences between {R} channel and {G, B} channel values can provide useful depth information for a given pixel.
As illustrated in this figure, differences in R and M values vary proportionately with pixel distances, as demonstrated by six 20 × 20 RoIs selected on the left image.
We exploit these inherent relationships and demonstrate that RMIā”{R, M=max{G,B}, I (intensity)} is a significantly better input space for visual learning pipelines of underwater monocular depth estimation models.
UDepth Project
Based on the wavelength-dependent attenuation constraints of the underwater domain, we design a domain projection step for pixel-wise coarse depth prediction.
We express the R-M relationship with the depth of the pixel with a linear approximator, then we find an efficient least-squared formulation by optimizing on the RGB-D training pairs.
We futher extend our domain projection step with guided filtering for fast coarse depth prediction. The domain projection achieves 51.5 FPS rates on single-board Jetson TX2s.
SDU-SfM Project
We further extend the linear domain projection idea above with non-linear domain projection for more fine-grained depth estimation. With depth-guided feature filtering and matching,
we formulate a 3D reconstruction pipeline for underwater imagery that reduces the dependency on the standard 2D feature matching and ensures a denser scene recovery.
Our proposed method SDU-SfM It uses pixel-wise depth information as a guide to enhance underwater images as well as to generate sparse to semi-dense point clouds at a low computational cost.
SDU-SfM offers over three times faster inference than existing algorithms and standard software packages (ColMap, Alice Vision)
for multi-view 3D reconstruction.
Paper
Publications
- B. Yu, J. Wu, and M. J. Islam. UDepth: Fast Monocular Depth Estimation for Visually-guided Underwater Robots. IEEE International Conference on Robotics and Automation (ICRA), 2023, London, UK. [project page pre-print code]
- J. Wu, B. Yu, and M. J. Islam. 3D Reconstruction of Underwater Scenes using Nonlinear Domain Projection. IEEE Conference on Artificial Intelligence (CAI), 2023, Santa Clara, CA, US. (Best paper award)